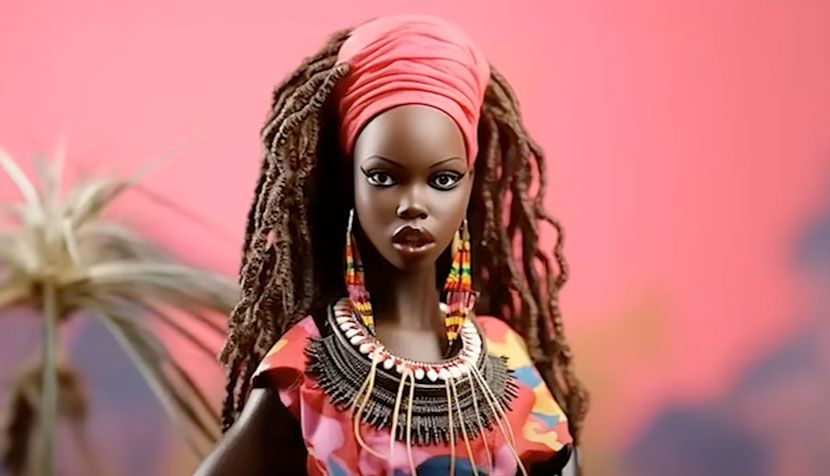
What
happened when artificial Intelligence (AI) was used to imagine Barbie
dolls in cultures around the world? Probably not what you’d expect for a
brand that has gained a massive following for breaking down barriers
and, more recently, advocating for social and cultural inclusion. In
fact, the resulting images were so offensive and caused such a backlash,
they had to be taken down.
How could this have possibly happened?
The answer lies in the fact that the rapid advancement of AI has unveiled a complex issue: bias. Bias in AI systems has the potential to reinforce societal inequalities and create unintended, detrimental consequences. And that’s exactly what happened when Buzzfeed published a now-deleted article on what AI thinks Barbie would look like if she were from various countries around the world. The results contained extreme colorist and racist depictions. In the opening paragraphs of the post, BuzzFeed wrote that the Barbies “reveal biases and stereotypes that currently exist within AI models,” noting that they are “not meant to be seen as accurate or full depictions of human experience.” However, that disclaimer did not stop people from expressing outrage and criticizing the outlet for creating something highly offensive and harmful.
AI image generators intensify bias
AI image generators, in particular, are becoming increasingly popular due to their impressive results and ease of use. However, these generators often introduce harmful biases related to gender, race, age and skin color that are more extreme than real-world biases. Researchers found that images that were generated for higher-paying professions tended to be associated with lighter skin tones and men, while lower-paying jobs were linked to darker skin tones and women, magnifying racial and gender biases. This phenomenon is known as representational harm, which reinforces stereotypes and inequalities. Because the biased images generated by AI systems are fed back into datasets, a feedback loop is created that intensifies existing biases.
The quest for fair representation
Resolving this problem involves deep philosophical questions about bias and fairness because defining what constitutes fair representation is tricky. While self-regulation by tech companies might not be sufficient, governments could potentially regulate AI technologies to mitigate representational harm. Establishing oversight bodies, imposing standards on training datasets and regulating algorithm updates are potential measures. However, the timing of these regulations is crucial, as acting too early or too late could lead to ineffective outcomes. Learn more in the video below, which was inspired by a London Interdisciplinary School student’s end-of-first-year project entitled “Beyond the Hype: Understanding Bias in AI and its Far-Reaching Consequences.” (You can also read more about the issue of bias in AI by reading this additional blog article from SunShower Learning.)
How could this have possibly happened?
The answer lies in the fact that the rapid advancement of AI has unveiled a complex issue: bias. Bias in AI systems has the potential to reinforce societal inequalities and create unintended, detrimental consequences. And that’s exactly what happened when Buzzfeed published a now-deleted article on what AI thinks Barbie would look like if she were from various countries around the world. The results contained extreme colorist and racist depictions. In the opening paragraphs of the post, BuzzFeed wrote that the Barbies “reveal biases and stereotypes that currently exist within AI models,” noting that they are “not meant to be seen as accurate or full depictions of human experience.” However, that disclaimer did not stop people from expressing outrage and criticizing the outlet for creating something highly offensive and harmful.
AI image generators intensify bias
AI image generators, in particular, are becoming increasingly popular due to their impressive results and ease of use. However, these generators often introduce harmful biases related to gender, race, age and skin color that are more extreme than real-world biases. Researchers found that images that were generated for higher-paying professions tended to be associated with lighter skin tones and men, while lower-paying jobs were linked to darker skin tones and women, magnifying racial and gender biases. This phenomenon is known as representational harm, which reinforces stereotypes and inequalities. Because the biased images generated by AI systems are fed back into datasets, a feedback loop is created that intensifies existing biases.
The quest for fair representation
Resolving this problem involves deep philosophical questions about bias and fairness because defining what constitutes fair representation is tricky. While self-regulation by tech companies might not be sufficient, governments could potentially regulate AI technologies to mitigate representational harm. Establishing oversight bodies, imposing standards on training datasets and regulating algorithm updates are potential measures. However, the timing of these regulations is crucial, as acting too early or too late could lead to ineffective outcomes. Learn more in the video below, which was inspired by a London Interdisciplinary School student’s end-of-first-year project entitled “Beyond the Hype: Understanding Bias in AI and its Far-Reaching Consequences.” (You can also read more about the issue of bias in AI by reading this additional blog article from SunShower Learning.)